Have you ever taken a COVID test with positive results but later found out you didn’t have COVID? Or tested negative, but later discovered you actually did have it?
These errors are called Type I and Type II errors. They can lead to:
Unnecessary actions or worry
Missed opportunities or unresolved issues
Type I and Type II errors directly affect how accurate and reliable data-driven decisions are.
What are data-driven decisions?
Data-driven decisions are made in various fields, such as scientific research, data analytics, and machine learning.
For example, deciding:
Is a certain treatment recommended?
Will a potential business opportunity be successful?
Is a certain transaction a fraud?
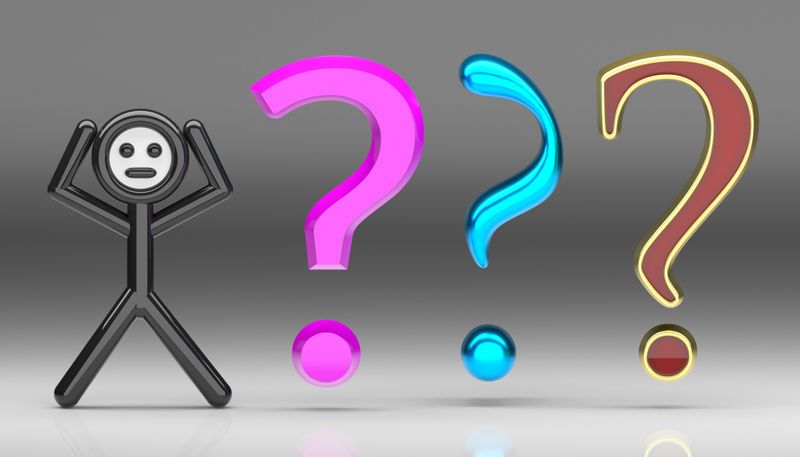
How are data-driven decisions made?
Hypothesis testing is a way to use data to decide if something is true or not.
You start with a null hypothesis which says the parameter checked has no effect. For example: “A certain treatment is not recommended.” Then, based on the data, you either:
Find an effect,which means you reject the null hypothesis.
Don't find an effect, which means you don't reject it.
1. Eureka! (Type I error)
You found an effect in the data and successfully rejected the null hypothesis. For instance, the sample of people who received the tested treatment did show better recovery.
But...sorry to cool off the excitement. There is still a certain chance that this conclusion is a mistake — meaning the effect found isn't related to the checked parameter, or the detected event was misidentified. This mistake is called a "false positive" and is known as a Type I error.
2. No breakthrough (Type II error)
The other scenario would be that, according to the data, there is no effect, or an event is not likely to be happening. You have failed in rejecting the null hypothesis. For example, people who received the tested treatment didn't show better recovery.
Also in this case there is a chance of a mistake, and the effect or the event might be missed. This type of error is called a "false negative", also known as a Type II error.
Test Your Knowledge
Which of these statements represents a Type I error? Select all that apply:
Scientists concluded that a certain medication is not causing heart problems when, in fact, it is.
Scientists concluded that a certain medication is causing heart problems when, in fact, it is not.
The model predicted that a transaction is fraudulent when, in fact, it is not.
The model predicted that a transaction is not fraudulent when, in fact, it is.
Quiz
Which represents a Type I error?
Take Action
To learn more about Type I and Type II errors:
Your feedback matters to us.
This Byte helped me better understand the topic.