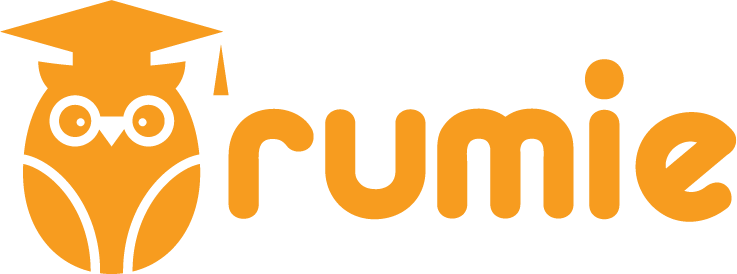
This logo isn't an ad or affiliate link. It's an organization that shares in our mission, and empowered the authors to share their insights in Byte form.
Rumie vets Bytes for compliance with our
Standards.
The organization is responsible for the completeness and reliability of the content.
Learn more
about how Rumie works with partners.
These two types of errors pop up everywhere, from medical tests to business decisions and even courtroom verdicts. Let’s break down the difference between Type I vs. Type II errors in statistics.
Understanding Null Hypothesis
In an ideal world, we can ask everyone and collect all the data we need. But realistically, researchers rely on sample sizes. The smaller the sample size, it's likely that there will be a faulty result.
Back to the hospital example, a medicine might work for some patients. However, some may be allergic to the medication. We need to test to make sure it's safe.
What is a Type I Error? (False Positive)
A Type I error happens when we reject a null hypothesis, a hypothesis that is in fact true. In simple terms, it’s when you think something is happening when it actually isn’t.
Example:
A pregnancy test wrongly says someone is pregnant when they’re not.
A fire alarm goes off when there’s no fire.
In statistics, professionals use numerous tests such as "t-statistics" or "chi-square" to determine how likely a conclusion will be a Type I error (a false alarm). Typically, a value below 5% can be considered acceptable for general research, but not for medical drugs
Which of the following situations describe a Type I error (false positive)?
A. A bank’s fraud detection system mistakenly flags a legitimate transaction as fraud and blocks the customer’s credit card.
B. A self-driving car’s system wrongly identifies a plastic bag on the road as a large obstacle and slams the brakes.
C. A job recruiter ignores a highly qualified candidate, thinking they wouldn’t be a good fit when they actually would have been perfect.
D. A professor incorrectly accuses a student of cheating on a test when they didn’t.
Quiz
Which situations describe a Type I error (false positive)? Select all that apply:
A job recruiter ignoring a qualified candidate isn't a false alarm (Type 1 error) but a wrong assumption (Type 2 error).
What is a Type II Error? (False Negative)
A Type II error happens when we fail to reject a false hypothesis. This means we miss something that’s actually there.
Example:
A test fails to detect a disease when the person actually has it.
A security scanner at the airport misses a hidden weapon.
Researchers may commonly use the Statistical Power Test to see how likely a conclusion will be a Type II error. This is important because missing a deadly disease could mean life or death in some situations.
Which of the following situations describe a Type II error (false negative)?
A. A medical test wrongly detects a disease in a healthy patient.
B. A smoke detector fails to go off even though there is a real fire.
C. A new plagiarism detection software fails to flag a clearly plagiarized essay.
D. A hiring manager overlooks a brilliant candidate, assuming they wouldn’t perform well in the role when they actually would have excelled.
Quiz
Which situations describe a Type II error (false negative)? Select all that apply:
A wrong medical detection would be Type I error (a false positive), not Type II.
Key Differences Between Type I vs. Type II Errors
A Type I error (false positive) means detecting something that isn’t there —like an unnecessary panic or overreaction.
A Type II error (false negative) means failing to detect something that is actually there — like overlooking an important warning sign.
A Type I error is the alarm going off when there’s no fire.
A Type II error is a fire breaking out, but the alarm doesn’t sound.
Think of Type I vs. Type II errors like fire alarms:
Quiz
A company launches a new product based on customer survey data, believing customers will love it. But after release, sales are terrible, proving their assumption wrong. What type of error is this?
This is a Type I error because the company assumed something was true when it wasn’t.
Why Do These Errors Matter?
In medicine, we usually prefer to reduce Type II errors because missing a disease can be life-threatening. That’s why tests tend to err on the side of caution.
In criminal justice, we want to minimize Type I errors — it’s worse to convict an innocent person than to let a guilty one go free.
In business, a Type I error could mean wasting money on a bad idea, while a Type II error could mean missing out on a breakthrough opportunity.
In statistics, the likelihood of Type I error is referred to as alpha (α). If we set α at 5%, we're less likely to miss something that happens (Type II error).
But if we decide to set the test conservatively at 0.5% for α, we probably won't commit Type I error (false alarm). However, it also means we might miss something that's there.
Take Action
Now that you've learned about the important difference between Type I vs. Type II errors, try out some activities!
This Byte has been authored by
Changsoo Park
Learning & Development Professional
M.Ed.